Prochlorococcus Metapangenome - Anvi'o Server
anvi'oは様々な解析方法や表現方法をサポートするマルチオミクス解析パッケージである。その機能の1つに、パンゲノムやメタゲノム(binned.fasta)のgenomic ANIを総当たりで計算し、 anvi'oマップにヒートマップのレイヤーとして表示する機能がある。ここではANI計算を行なってANIヒートマップレイヤー付きanvi'oマップを描く手順をまとめておきます。
マニュアル
インストール
公式dockerイメージを使ってubuntu18.04LTS上でテストした。
本体 Github
依存が多いので、condaだと依存チェックに異常な時間がかかる。dockerを使うと簡単。
#docker (dockerhub) (link)
#latest (v6)
docker pull meren/anvio:latest
インストールチェック
> anvi-self-test --suite pangenomics
help
> anvi-gen-contigs-database -h
> anvi-gen-contigs-database -h
usage: anvi-gen-contigs-database [-h] -f FASTA [-n PROJECT_NAME]
[-o DB_FILE_PATH] [--description TEXT_FILE]
[-L INT] [-K INT] [--skip-gene-calling]
[--prodigal-translation-table INT]
[--external-gene-calls GENE-CALLS]
[--ignore-internal-stop-codons]
[--skip-mindful-splitting]
Generate a new anvi'o contigs database.
optional arguments:
-h, --help show this help message and exit
MANDATORY INPUTS:
Things you really need to provide to be in business.
-f FASTA, --contigs-fasta FASTA
The FASTA file that contains reference sequences you
mapped your samples against. This could be a reference
genome, or contigs from your assembler. Contig names
in this file must match to those in other input files.
If there is a problem anvi'o will gracefully complain
about it.
-n PROJECT_NAME, --project-name PROJECT_NAME
Name of the project. Please choose a short but
descriptive name (so anvi'o can use it whenever she
needs to name an output file, or add a new table in a
database, or name her first born).
OPTIONAL INPUTS:
Things you may want to tweak.
-o DB_FILE_PATH, --output-db-path DB_FILE_PATH
Output file path for the new database.
--description TEXT_FILE
A plain text file that contains some description about
the project. You can use Markdwon syntax. The
description text will be rendered and shown in all
relevant interfaces, including the anvi'o interactive
interface, or anvi'o summary outputs.
-L INT, --split-length INT
Anvi'o splits very long contigs into smaller pieces,
without actually splitting them for real. These
'virtual' splits improve the efficacy of the
visualization step, and changing the split size gives
freedom to the user to adjust the resolution of their
display when necessary. The default value is (20000).
If you are planning to use your contigs database for
metagenomic binning, we advise you to not go below
10,000 (since the lower the split size is, the more
items to show in the display, and decreasing the split
size does not really help much to binning). But if you
are thinking about using this parameter for ad hoc
investigations other than binning, you should ignore
our advice, and set the split size as low as you want.
If you do not want your contigs to be split, you can
set the split size to '0' or any other negative
integer (lots of unnecessary freedom here, enjoy!).
-K INT, --kmer-size INT
K-mer size for k-mer frequency calculations. The
default k-mer size for composition-based analyses is
4, historically. Although tetra-nucleotide frequencies
seem to offer the the sweet spot of sensitivity,
information density, and manageable number of
dimensions for clustering approaches, you are welcome
to experiment (but maybe you should leave it as is for
your first set of analyses).
--skip-mindful-splitting
By default, anvi'o attempts to prevent soft-splitting
large contigs by cutting proper gene calls to make
sure a single gene is not broken into multiple splits.
This requires a careful examination of where genes
start and end, and to find best locations to split
contigs with respect to this information. So, when the
user asks for a split size of, say, 1,000, it serves
as a mere suggestion. When this flag is used, anvi'o
does what the user wants and creates splits at desired
lengths (although some functionality may become
unavailable for the projects that rely on a contigs
database that is initiated this way).
GENES IN CONTIGS:
Expert thingies.
--skip-gene-calling By default, generating an anvi'o contigs database
includes the identification of open reading frames in
contigs by running a bacterial gene caller. Declaring
this flag will by-pass that process. If you prefer,
you can later import your own gene calling results
into the database.
--prodigal-translation-table INT
This is a parameter to pass to the Prodigal for a
specific translation table. This parameter corresponds
to the parameter `-g` in Prodigal, the default value
of which is 11 (so if you do not set anything, it will
be set to 11 in Prodigal runtime. Please refer to the
Prodigal documentation to determine what is the right
translation table for you if you think you need it.)
--external-gene-calls GENE-CALLS
A TAB-delimited file to utilize external gene calls.
The file must have these columns: 'gene_callers_id' (a
unique integer number for each gene call, start from
1), 'contig' (the contig name the gene call is found),
'start' (start position, integer), 'stop' (stop
position, integer), 'direction' (the direction of the
gene open reading frame; can be 'f' or 'r'), 'partial'
(whether it is a complete gene call, or a partial one;
must be 1 for partial calls, and 0 for complete
calls), 'source' (the gene caller), and 'version' (the
version of the gene caller, i.e., v2.6.7 or v1.0). An
example file can be found via the URL
https://bit.ly/2qEEHuQ
--ignore-internal-stop-codons
This is only relevant when you have an external gene
calls file. If anvi'o figures out that your custom
gene calls result in amino acid sequences with stop
codons in the middle, it will complain about it. You
can use this flag to tell anvi'o to don't check for
internal stop codons, EVEN THOUGH IT MEANS THERE IS
MOST LIKELY SOMETHING WRONG WITH YOUR EXTERNAL GENE
CALLS FILE. Anvi'o will understand that sometimes we
don't want to care, and will not judge you. Instead,
it will replace every stop codon residue in the amino
acid sequence with an 'X' character. Please let us
know if you used this and things failed, so we can
tell you that you shouldn't have really used it if you
didn't like failures at the first place (smiley).
> anvi-gen-genomes-storage -h
> anvi-gen-genomes-storage -h
usage: anvi-gen-genomes-storage [-h] [-e FILE_PATH] [-i FILE_PATH]
[--gene-caller GENE-CALLER] -o GENOMES_STORAGE
Create a genome storage from internal or external genomes for a pan genome
analysis.
optional arguments:
-h, --help show this help message and exit
EXTERNAL GENOMES:
External genomes listed as anvi'o contigs databases. As in, you have one
or more genomes say from NCBI you want to work with, and you created an
anvi'o contigs database for each one of them.
-e FILE_PATH, --external-genomes FILE_PATH
A two-column TAB-delimited flat text file that lists
anvi'o contigs databases. The first item in the header
line should read 'name', and the second should read
'contigs_db_path'. Each line in the file should
describe a single entry, where the first column is the
name of the genome (or MAG), and the second column is
the anvi'o contigs database generated for this genome.
INTERNAL GENOMES:
Genome bins stored in an anvi'o profile databases as collections.
-i FILE_PATH, --internal-genomes FILE_PATH
A five-column TAB-delimited flat text file. The header
line must contain these columns: 'name', 'bin_id',
'collection_id', 'profile_db_path', 'contigs_db_path'.
Each line should list a single entry, where 'name' can
be any name to describe the anvi'o bin identified as
'bin_id' that is stored in a collection.
PRO STUFF:
Things you may not have to change. But you never know (unless you read the
help).
--gene-caller GENE-CALLER
The gene caller to utilize. Anvi'o supports multiple
gene callers, and some operations (including this one)
requires an explicit mentioning of which one to use.
The default is 'prodigal', but it will not be enough
if you if you were a rebel and have used `--external-
gene-callers` or something.
OUTPUT:
Give it a nice name. Must end with '-GENOMES.db'. This is primarily due to
the fact that there are other .db files used throughout anvi'o and it
would be better to distinguish this very special file from them.
-o GENOMES_STORAGE, --output-file GENOMES_STORAGE
File path to store results.
> anvi-pan-genome -h
> anvi-pan-genome -h
WARNING
===============================================
If you publish results from this workflow, please do not forget to cite DIAMOND
(doi:10.1038/nmeth.3176), unless you use it with --use-ncbi-blast flag, and MCL
(http://micans.org/mcl/ and doi:10.1007/978-1-61779-361-5_15)
usage: anvi-pan-genome [-h] -g GENOMES_STORAGE [-G GENOME_NAMES]
[--skip-alignments] [--skip-homogeneity]
[--quick-homogeneity] [--align-with ALIGNER]
[--exclude-partial-gene-calls] [--use-ncbi-blast]
[--minbit MINBIT] [--mcl-inflation INFLATION]
[--min-occurrence NUM_OCCURRENCE]
[--min-percent-identity PERCENT] [--sensitive]
[-n PROJECT_NAME] [--description TEXT_FILE]
[-o PAN_DB_DIR] [-W] [-T NUM_THREADS]
[--skip-hierarchical-clustering]
[--enforce-hierarchical-clustering]
[--distance DISTANCE_METRIC] [--linkage LINKAGE_METHOD]
A DIAMOND and MCL-based anvi'o workflow for pangenomics. You provide genomes
from anywhere (whether they are external genomes, or anvi'o genome bins in
collections), and it gives you back a pangenome analysis.
optional arguments:
-h, --help show this help message and exit
GENOMES:
The very fancy genomes storage file. This file is generated by the program
`anvi-genomes-storage`. Please see the online tutorial on pangenomic
workflow if you don't know how to generate one.
-g GENOMES_STORAGE, --genomes-storage GENOMES_STORAGE
Anvi'o genomes storage file
-G GENOME_NAMES, --genome-names GENOME_NAMES
Genome names to 'focus'. You can use this parameter to
limit the genomes included in your analysis. You can
provide these names as a comma-separated list of
names, or you can put them in a file, where you have a
single genome name in each line, and provide the file
path.
PARAMETERS:
Important stuff Tom never pays attention (but you should).
--skip-alignments By default, anvi'o attempts to align amino acid
sequences in each gene cluster using multiple sequnce
alignment via muscle. You can use this flag to skip
that step and be upset later.
--skip-homogeneity By default, anvi'o attempts to calculate homogeneity
values for every gene cluster, given that they are
aligned. You can use this flag to have anvi'o skip
homogeneity calculations. Anvi'o will ignore this flag
if you decide to skip alignments
--quick-homogeneity By default, anvi'o will use a homogeneity algorithm
that checks for horizontal and vertical geometric
homogeneity (along with functional). With this flag,
you can tell anvi'o to skip horizontal geometric
homogeneity calculations. It will be less accurate but
quicker. Anvi'o will ignore this flag if you skip
homogeneity calculations or alignments all together.
--align-with ALIGNER The multiple sequence alignment program to use when
multiple sequence alignment is necessary. To see all
available options, use the flag `--list-aligners`.
--exclude-partial-gene-calls
By default, anvi'o includes all partial gene calls
from the analysis, which, in some cases, may inflate
the number of gene clusters identified and introduce
extra heterogeneity within those gene clusters. Using
this flag, you can request anvi'o to exclude partial
gene calls from the analysis (whether a gene call is
partial or not is an information that comes directly
from the gene caller used to identify genes during the
generation of the contigs database).
--use-ncbi-blast This program uses DIAMOND by default, however, if you
like, you can use good ol' blastp from NCBI instead.
--minbit MINBIT The minimum minbit value. The minbit heuristic
provides a mean to set a to eliminate weak matches
between two amino acid sequences. We learned it from
ITEP (Benedict MN et al, doi:10.1186/1471-2164-15-8),
which is a comprehensive analysis workflow for
pangenomes, and decided to use it in the anvi'o
pangenomic workflow, as well. Briefly, If you have two
amino acid sequences, 'A' and 'B', the minbit is
defined as 'BITSCORE(A, B) / MIN(BITSCORE(A, A),
BITSCORE(B, B))'. So the minbit score between two
sequences goes to 1 if they are very similar over the
entire length of the 'shorter' amino acid sequence,
and goes to 0 if (1) they match over a very short
stretch compared even to the length of the shorter
amino acid sequence or (2) the match betwen sequence
identity is low. The default is 0.5.
--mcl-inflation INFLATION
MCL inflation parameter, that defines the sensitivity
of the algorithm during the identification of the gene
clusters. More information on this parameter and it's
effect on cluster granularity is here:
(http://micans.org/mcl/man/mclfaq.html#faq7.2). The
default is 2.
--min-occurrence NUM_OCCURRENCE
Do you not want singletons?\ You don't? Well, this
parameter will help you get rid of them (along with
doubletons, if you want). Anvi'o will remove gene
clusters that occur less than the number you set using
this parameter from the analysis. The default is 1,
which means everything will be kept. If you want to
remove singletons, set it to 2, if you want to remove
doubletons as well, set it to 3, and so on.
--min-percent-identity PERCENT
Minimum percent identity between the two amino acid
sequences for them to have an edge for MCL analysis.
This value will be used to filter hits from Diamond
search results. Because percent identity is not a
predictor of a good match (since it does not
communicate many other important factors such as the
alignment length between the two sequences and its
proportion to the entire length of those involved), we
suggest you rely on 'minbit' parameter. But you know
what? Maybe you shouldn't listen to anyone, and
experiment on your own! The default is 0 percent.
--sensitive DIAMOND sensitivity. With this flag you can instruct
DIAMOND to be 'sensitive', rather than 'fast' during
the search. It is likely the search will take
remarkably longer. But, hey, if you are doing it for
your final analysis, maybe it should take longer and
be more accurate. This flag is only relevant if you
are running DIAMOND.
OTHERS:
Sweet parameters of convenience.
-n PROJECT_NAME, --project-name PROJECT_NAME
Name of the project. Please choose a short but
descriptive name (so anvi'o can use it whenever she
needs to name an output file, or add a new table in a
database, or name her first born).
--description TEXT_FILE
A plain text file that contains some description about
the project. You can use Markdwon syntax. The
description text will be rendered and shown in all
relevant interfaces, including the anvi'o interactive
interface, or anvi'o summary outputs.
-o PAN_DB_DIR, --output-dir PAN_DB_DIR
Directory path for output files
-W, --overwrite-output-destinations
Overwrite if the output files and/or directories
exist.
-T NUM_THREADS, --num-threads NUM_THREADS
Maximum number of threads to use for multithreading
whenever possible. Very conservatively, the default is
1. It is a good idea to not exceed the number of CPUs
/ cores on your system. Plus, please be careful with
this option if you are running your commands on a SGE
--if you are clusterizing your runs, and asking for
multiple threads to use, you may deplete your
resources very fast.
ORGANIZING GENE CLUSTERs:
These are stuff that will change the clustering dendrogram of your gene
clusters.
--skip-hierarchical-clustering
Anvi'o attempts to generate a hierarchical clustering
of your gene clusters once it identifies them so you
can use `anvi-display-pan` to play with it. But if you
want to skip this step, this is your flag.
--enforce-hierarchical-clustering
If you want anvi'o to try to generate a hierarchical
clustering of your gene clusters even if the number of
gene clusters exceeds its suggested limit for
hierarchical clustering, you can use this flag to
enforce it. Are you are a rebel of some sorts? Or did
computers made you upset? Express your anger towards
machine using this flag.
--distance DISTANCE_METRIC
The distance metric for the clustering of gene
clusters. If you do not use this flag, the default
distance metric will be used for each clustering
configuration which is "euclidean".
--linkage LINKAGE_METHOD
The same story with the `--distance`, except, the
system default for this one is ward.
> anvi-compute-genome-similarity -h
> anvi-compute-genome-similarity -h
usage: anvi-compute-genome-similarity [-h] [-i FILE_PATH] [-e FILE_PATH]
[-f FASTA_TEXT_FILE] -o DIR_PATH
[-p PAN_DB]
[--program {pyANI,fastANI,sourmash}]
[--fastani-kmer-size FASTANI_KMER_SIZE]
[--fragment-length FRAGMENT_LENGTH]
[--min-num-fragments MIN_NUM_FRAGMENTS]
[--method {ANIm,ANIb,ANIblastall,TETRA}]
[--min-alignment-fraction NUM]
[--significant-alignment-length INT]
[--min-full-percent-identity FULL_PERCENT_IDENTITY]
[--kmer-size INT] [--scale INT]
[--distance DISTANCE_METRIC]
[--linkage LINKAGE_METHOD]
[-T NUM_THREADS] [--just-do-it]
[--log-file FILE_PATH]
Export sequences from sequence sources and compute a similarity metric (e.g.
ANI). If a Pan Database is given anvi'o will write computed output to misc
data tables of Pan Database.
optional arguments:
-h, --help show this help message and exit
INPUT OPTIONS:
Tell anvi'o what you want.
-i FILE_PATH, --internal-genomes FILE_PATH
A five-column TAB-delimited flat text file. The header
line must contain these columns: 'name', 'bin_id',
'collection_id', 'profile_db_path', 'contigs_db_path'.
Each line should list a single entry, where 'name' can
be any name to describe the anvi'o bin identified as
'bin_id' that is stored in a collection.
-e FILE_PATH, --external-genomes FILE_PATH
A two-column TAB-delimited flat text file that lists
anvi'o contigs databases. The first item in the header
line should read 'name', and the second should read
'contigs_db_path'. Each line in the file should
describe a single entry, where the first column is the
name of the genome (or MAG), and the second column is
the anvi'o contigs database generated for this genome.
-f FASTA_TEXT_FILE, --fasta-text-file FASTA_TEXT_FILE
A two-column TAB-delimited file that lists multiple
FASTA files to import for analysis. If using for
`anvi-dereplicate-genomes` or `anvi-compute-distance`,
each FASTA is assumed to be a genome. The first item
in the header line should read 'name', and the second
item should read 'path'. Each line in the field should
describe a single entry, where the first column is the
name of the FASTA file or corresponding sequence, and
the second column is the path to the FASTA file
itself.
OUTPUT OPTIONS:
Tell anvi'o where to store your results.
-o DIR_PATH, --output-dir DIR_PATH
Directory path for output files
-p PAN_DB, --pan-db PAN_DB
This is totally optional, but very useful when
applicable. If you are running this for genomes for
which you already have an anvi'o pangeome, then you
can show where the pan database is and anvi'o would
automatically add the results into the misc data
tables of your pangenome. Those data can then be shown
as heatmaps on the pan interactive interface through
the 'layers' tab.
Program:
Tell anvi'o which similarity program to run.
--program {pyANI,fastANI,sourmash}
Tell anvi'o which program to run to process genome
similarity. For ANI, you should either use pyANI or
fastANI. If accuracy is paramount (for example,
distinguishing things less than 1 percent different),
or for dealing with genomes < 80 percent similar,
pyANI is what we recommend. However, fastANI is much
faster. If you for some reason want to use mash
similarity, you can use sourmash, but its really not
intended for genome comparisons. If you don't choose
anything here, anvi'o will reluctantly set the program
to pyANI, but you really should be the one who is on
top of these things.
fastANI Settings:
Tell anvi'o to tell fastANI what settings to set. Only if `--program` is
set to `pyANI`
--fastani-kmer-size FASTANI_KMER_SIZE
Choose a kmer. The default is 16.
--fragment-length FRAGMENT_LENGTH
Choose a fragment length. The default is 3000.
--min-num-fragments MIN_NUM_FRAGMENTS
Choose the minimum number of fragment lengths to that
can can be trusted. The default is 50.
pyANI Settings:
Tell anvi'o to tell pyANI what method you wish to use and what settings to
set. Only if `--program` is set to `pyANI`
--method {ANIm,ANIb,ANIblastall,TETRA}
Method for pyANI. The default is ANIb. You must have
the necessary binary in path for whichever method you
choose. According to the pyANI help for v0.2.7 at
https://github.com/widdowquinn/pyani, the method
'ANIm' uses MUMmer (NUCmer) to align the input
sequences. 'ANIb' uses BLASTN+ to align 1020nt
fragments of the input sequences. 'ANIblastall': uses
the legacy BLASTN to align 1020nt fragments Finally,
'TETRA': calculates tetranucleotide frequencies of
each input sequence
--min-alignment-fraction NUM
In some cases you may get high raw ANI estimates
(percent identity scores) between two genomes that
have little to do with each other simply because only
a small fraction of their content may be aligned. This
filter will set all ANI scores between two genomes to
0 if the alignment fraction is less than you deem
trustable. When you set a value, anvi'o will go
through the ANI results, and set percent identity
scores between two genomes to 0 if the alignment
fraction *between either of them* is less than the
parameter described here. The default is 0.
--significant-alignment-length INT
So --min-alignment-fraction discards any hit that is
coming from alignments that represent shorter
fractions of genomes, but what if you still don't want
to miss an alignment that is longer than an X number
of nucleotides regardless of what fraction of the
genome it represents? Well, this parameter is to
recover things that may be lost due to --min-
alignment-fraction parameter. Let's say, if you set
--min-alignment-fraction to '0.05', and this parameter
to '5000', anvi'o will keep hits from alignments that
are longer than 5000 nts, EVEN IF THEY REPRESENT less
than 5 percent of a given genome pair. Basically if
--min-alignment-fraction is your shield to protect
yourself from incoming garbage, --significant-
alignment-length is your chopstick to pick out those
that may be interesting, and you are a true warrior
here.
--min-full-percent-identity FULL_PERCENT_IDENTITY
In some cases you may get high raw ANI estimates
(percent identity scores) between two genomes that
have little to do with each other simply because only
a small fraction of their content may be aligned. This
can be partly alleviated by considering the *full*
percent identity, which includes in its calculation
regions that did not align. For example, if the
alignment is a whopping 97 percent identity but only 8
percent of the genome aligned, the *full* percent
identity is 0.970 * 0.080 = 0.078 OR 7.8 percent.
*full* percent identity is always included in the
report, but you can also use it as a filter for other
metrics, such as percent identity. This filter will
set all ANI measures between two genomes to 0 if the
*full* percent identity is less than you deem
trustable. When you set a value, anvi'o will go
through the ANI results, and set all ANI measures
between two genomes to 0 if the *full* percent
identity *between either of them* is less than the
parameter described here. The default is 0.
Sourmash Settings:
Tell anvi'o to tell sourmash what settings to set. Only if `--program` is
set to `sourmash`
--kmer-size INT Set the k-mer size for mash similarity checks. We
found 13 in almost all cases correlates best with
alignment-based ANI.
--scale INT Set the compression ratio for fasta signature file
computations. The default is 1000. Smaller ratios
decrease sensitivity, while larger ratios will lead to
large fasta signatures.
HIERARCHICAL CLUSTERING:
anvi-compute-genome-similarity outputs similarity matrix files, which can
be clustered into nice looking dendrograms to display the relationships
between genomes nicely (in the anvi'o interface and elsewhere). Here you
can set the distance metric and the linkage algorithm for that.
--distance DISTANCE_METRIC
The distance metric for the hierarchical clustering.
The default is "euclidean".
--linkage LINKAGE_METHOD
The linkage method for the hierarchical clustering.
The default is "ward".
OTHER IMPORTANT STUFF:
Yes. You're almost done.
-T NUM_THREADS, --num-threads NUM_THREADS
Maximum number of threads to use for multithreading
whenever possible. Very conservatively, the default is
1. It is a good idea to not exceed the number of CPUs
/ cores on your system. Plus, please be careful with
this option if you are running your commands on a SGE
--if you are clusterizing your runs, and asking for
multiple threads to use, you may deplete your
resources very fast.
--just-do-it Don't bother me with questions or warnings, just do
it.
--log-file FILE_PATH File path to store debug/output messages.
実行方法
ここではパンゲノム解析を想定して進める。
1、FASTAファイルが’置いてある作業ディレクトリにてanvi'oのdockerイメージを立ち上げる。
docker run --rm -it -v `pwd`:`pwd` -w `pwd` -p 8080:8080 meren/anvio:latest
2、microbial genomeのFASTAファイルを対象にデータベースを作成する。この作業はゲノムごとに順番に行う必要があり時間がかかる。計算リソースが潤沢なら、バックグラウンドに回して並行処理することでスピードアップできる。
anvi-gen-contigs-database -f ghenome1.fna -o genome1.db -n 'genome1' &
anvi-gen-contigs-database -f ghenome2.fna -o genome2.db -n 'genome2' &
anvi-gen-contigs-database -f ghenome3.fna -o genome3.db -n 'genome3' &
anvi-gen-contigs-database -f ghenome4.fna -o genome4.db -n 'genome4' &
anvi-gen-contigs-database -f ghenome5.fna -o genome5.db -n 'genome5' &
anvi-gen-contigs-database -f ghenome6.fna -o genome6.db -n 'genome6' &
anvi-gen-contigs-database -f ghenome7.fna -o genome7.db -n 'genome7' &
anvi-gen-contigs-database -f ghenome8.fna -o genome8.db -n 'genome8' &
注意;FASTAファイルのヘッダやファイル名で割と一般的に使われるのが"-", "<space>", "-"などですが、これらはファイル内に存在してもファイル名に存在してもエラーを起こします。必ず置換しておいてください。アンダーバー”_”に置換しておけばエラーは起きません。また、"-n"で指定する名前は視覚化されるときに使われます。禁則文字に注意しつつ適切な名前をつけてください。
3、データベースを統合する。タブ区切りのリストファイルを与える必要がある。-nで指定した名前とdbファイル名が記載されたファイルになる。
list.txt
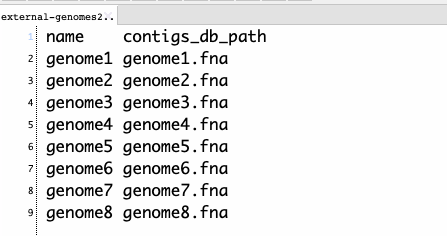
リストファイルとデータベース名を指定して実行する。
anvi-gen-genomes-storage -e list.txt -o PROCHLORO-GENOMES.db
統合されたデータベースPROCHLORO-GENOMES.dbが出力される。
4、anvi-pan-genomeプログラムを使ってパンゲノム解析を実行する。3の出力であるPROCHLORO-GENOMES.dbを指定する。
anvi-pan-genome -g PROCHLORO-GENOMES.db -n PROJECT -T 40
ディレクトリ PROJECT/ができ、ディレクトリ内にパンゲノムデータベースPROJECT-PAN.dbと関連ファイルが出力される。以降はPROJECT-PAN.dbを使う。
5、既にデータベースは作成されておりいつでも視覚化できるが、その前にANI計算をして視覚化時にヒートマップレイヤーを選択できるようにする。anvi-compute-genome-similarity コマンドを使う。このコマンドにはPyani(紹介)などの代表的な総当たりANI計算プログラムが組み込まれている。ANIの計算方法はPyaniのGIthub参照。
3で使ったリストファイルとANI計算方法を指定して実行する。
anvi-compute-genome-similarity -p PROCHLORO-GENOMES/PROJECT-PAN.db --program pyANI --method ANIm -T 40 --log-file log -e
list.txt -o ANI
-
--program {pyANI, fastANI, sourmash} Tell anvi'o which program to run to process genome similarity. For ANI, you should either use pyANI or fastANI. If accuracy is paramount (for example, distinguishing things less than 1 percent different), or for dealing with genomes < 80 percent similar, pyANI is what we recommend. However, fastANI is much faster. If you for some reason want to use mash similarity, you can use sourmash, but its really not intended for genome comparisons. If you don't choose anything here, anvi'o will reluctantly set the program to pyANI, but you really should be the one who is on top of these things.
-
--method {ANIm, ANIb, ANIblastall, TETRA} Method for pyANI. The default is ANIb. You must have the necessary binary in path for whichever method you choose. According to the pyANI help for v0.2.7 at https://github.com/widdowquinn/pyani, the method 'ANIm' uses MUMmer (NUCmer) to align the input sequences. 'ANIb' uses BLASTN+ to align 1020nt fragments of the input sequences. 'ANIblastall': uses the legacy BLASTN to align 1020nt fragments Finally, 'TETRA': calculates tetranucleotide frequencies of each input sequence
- -o Directory path for output files
- -p <PAN_DB> This is totally optional, but very useful when applicable. If you are running this for genomes for which you already have an anvi'o pangeome, then you can show where the pan database is and anvi'o would automatically add the results into the misc data tables of your pangenome. Those data can then be shown as heatmaps on the pan interactive interface through the 'layers' tab.
- -T Maximum number of threads to use for multithreading whenever possible. Very conservatively, the default is 1. It is a good idea to not exceed the number of CPUs / cores on your system. Plus, please be careful with this option if you are running your commands on a SGE --if you are clusterizing your runs, and asking for multiple threads to use, you may deplete your resources very fast.
- --log-file File path to store debug/output messages.
指定したディレクトリに総当たりANI計算結果やnewickファイルが出力される。データベースを指定してランしていれば、ANI計算結果は既にデータベースに組み込まれている。
6、視覚化する。
anvi-display-pan -p PROJECT1/PROJECT-PAN.db -g PROCHLORO-GENOMES.db
http://localhost:8080 にアクセスする。
レイヤータブでANIにチェックをつける。
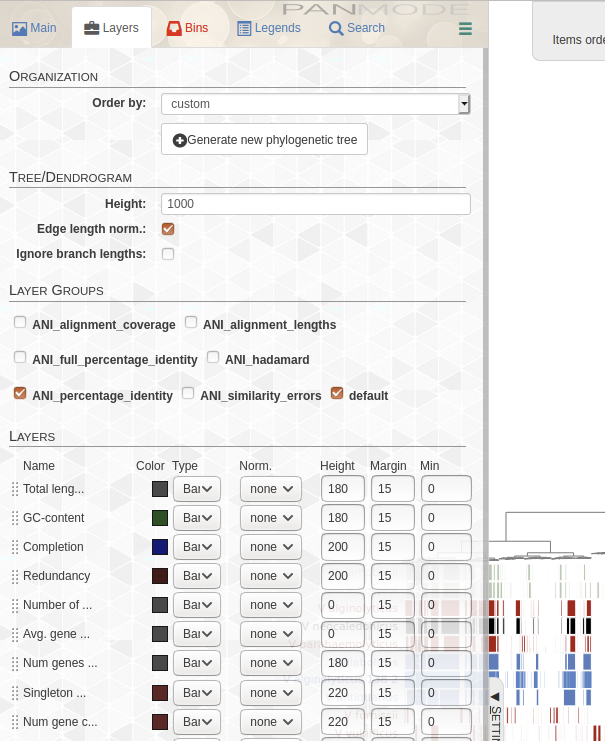
完成。右上にANIのヒートマップが追加された。
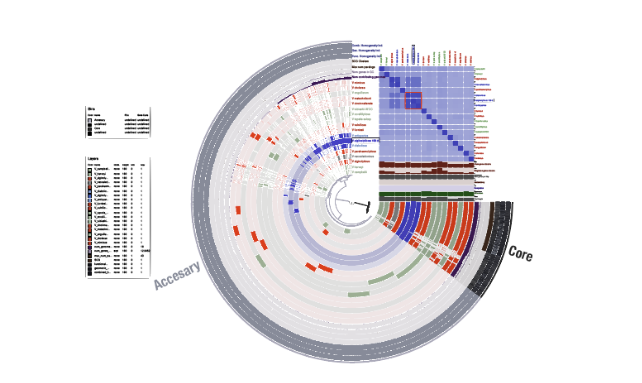
ヒートマップはリングの位置関係と揃っているように見えますが、完全には同期していないので注意してください。
マニュアルで完成例を見ることができます。
web server (pangenome)
anvi'o server
引用
Anvi'o: an advanced analysis and visualization platform for 'omics data
Eren AM, Esen ÖC, Quince C, Vineis JH, Morrison HG, Sogin ML, Delmont TO
PeerJ. 2015 Oct 8;3:e1319
関連